The application of Artificial Intelligence (AI) and Machine Learning (ML) in the domain of software testing will prove to be productive and rewarding and it will bring a whole new meaning to the software testing realm in terms of performance. AI in software testing and ML will be able to detect complex errors faster and carry out certain key testing activities in a streamlined and efficient manner.
In this article, you will get to know how machine learning and AI in software testing will bring a new dimension to software testing.
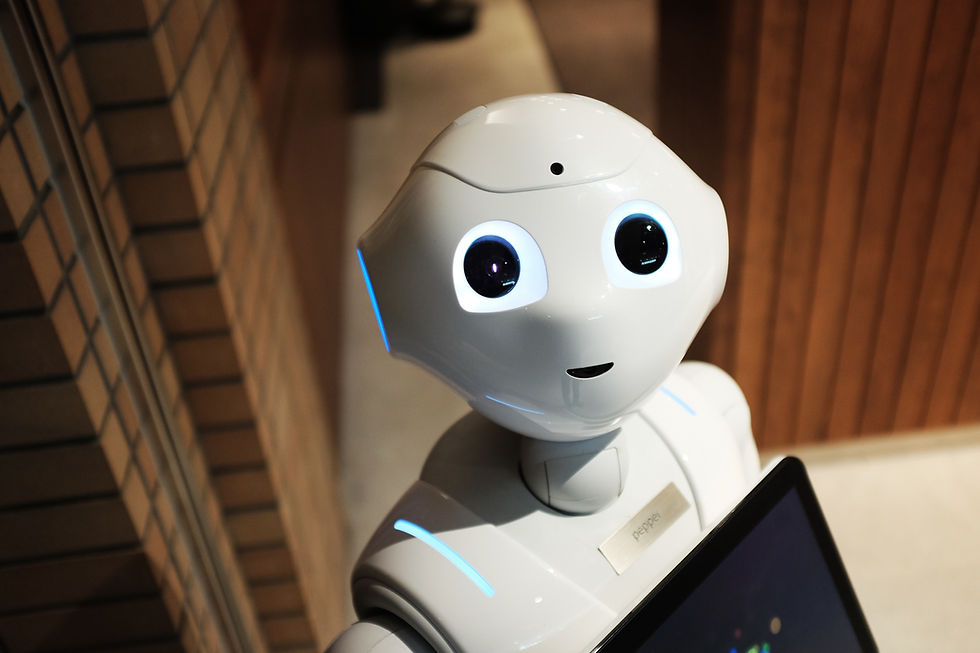
Following are the five factors that depict how AI in Software Testing and ML will bring a new dimension to software testing:
1. The testing process will be automated: When there are more tests that need to be tested, the process becomes more expensive and labor-intensive. Furthermore, when the application is being changed, the test code needs to be updated by the QA engineers. The maintenance part is streamlined when automation efforts are being put in. Even after changes have been made to the code, AI will evolve continually. As the bots are not fully encoded, they find new application functions by learning and adapting themselves.
When modifications are being identified by the AI platform, it estimates automatically whether it is defects of a new release or it is a new functionality. After the application code goes through every change, manual refinement is required for hard-coded test scripts as they are fragile.
2. More bugs are eliminated: There will be bugs in QA processes. In certain scenarios, the QA engineers despite trying their best to detect bugs will not be able to detect certain bugs that will go unnoticed. With the inclusion of an AI platform in testing, it will be able to detect unnoticed bugs due to its technological advantage. This information can be used by testers in order to decide whether there is a requirement for coding changes to prevent program errors.
Ongoing analysis of errors can be conducted by the AI platform in the QA scenarios.
3. Ignored bugs are focused upon: There will be negative consequences if ignored bugs are diverse and not dealt with accordingly. In a traditional testing scenario, if enough attention is not paid to the data management, then several ignored bugs can be received as a result. This will in turn dissatisfy the customer and thus the brand’s reputation can be spoilt.
When the Machine Learning approach is adopted, then more reliable testing outcomes can be expected. The time needed to detect bugs during software testing will also reduce to a great extent.
4. Client requirements can be easily forecasted: For any organization, it becomes quite obvious that by implementing certain specific processes and systems, there will be a good number of chances to get a notch higher than their competitors. In software testing scenarios, forecasting client requirements is considered to be a strategic and wise move.
Enterprises can be empowered through forecasting so that the customer data can be analyzed and the product and their respective features can be properly understood. All the above mentioned points can be easily solved by implementing the machine learning platform.
5. A strategic alternative for manual testing: There is a lot of time that will be consumed by performing manual testing and upon that manual testers have to work laboriously. With the inclusion of an AI platform, scripts can be written faster and large amounts of data sets can be easily analyzed. Log files can be sorted through the AI platform, which will in turn save time and the program correctness can be enhanced.
A complete view of alterations that need to be carried out by the QA engineers in terms of possible outcomes is provided by the AI platform.
Conclusion: If you are looking forward to implementing AI in software testing for your specific project, then do get connected with a premium software testing services company that will provide you tactical AI testing solutions that will be in line with your software development and software testing activities.
About the author: I am a technical content writer focused on writing technology specific articles. I strive to provide well-researched information on the leading market savvy technologies.
Bình luận